Artificial Intelligence (AI) is taking an increasingly central role in the logistics industry. You could call it a real AI craze. Every week new articles pop up preaching the technology and how it will become the solution for the ever growing complexity of the logistics industry. Additionally most articles and blog posts mention the same reports by Accenture and Forbes, which state bold claims like: “by the year 2035 productivity will have increased by more than 40% due to artificial intelligence”. Though persuasive, these numbers don’t tell us anything about the innovations artificial intelligence has produced for the logistics industry. For this reason I wanted to ask the real question, where has AI brought us so far?
Moreover, I’m specifically interested in the status of AI as enabler of the so-called physical internet. Already in 2016 it was ushered that AI was the start of “autonomous supply chains which will provide an opportunity for previously unimaginable levels of optimization”. The term autonomous supply chains is one of the fundamentals of the physical internet concept. Therefore, it is interesting to see if we are anywhere close to this Utopian view of self-governing supply chains.
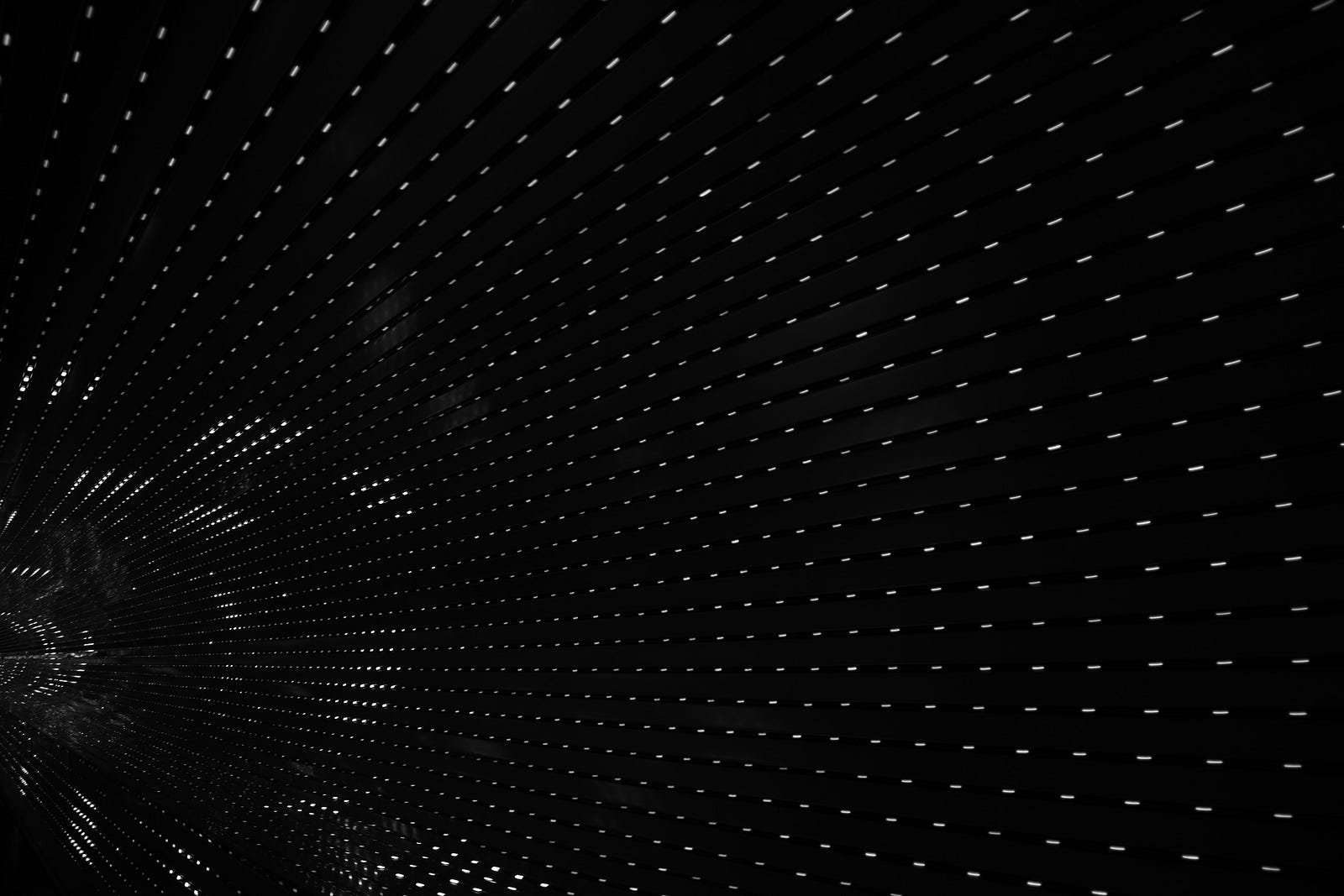
First steps towards physical internet
There’s a wide array of use cases for artificial intelligence in logistics. Overall the use cases can be grouped into: automated warehouses and robotics, document flow and natural language processing (NLP),predictive maintenance, and autonomous vehicles.
All of these cases are extremely interesting and prove a lot of value in our path to more efficient supply chains. However, I want to focus on the use cases that could be regarded as the first steps toward the well-discussed topic of the physical internet.The most important aspect of this concept in my opinion is the creation of a smart system that answers the most basic question one can ask in regards to transportation: “What’s the best way to get from A to B?”.
Answering this question through software isn’t new, it’s called route optimization and this use case has long proven its business value. Many companies have arisen to use AI and machine learning to create the ideal algorithm to find the fastest, cheapest, most efficient route. Some examples of companies leveraging AI to analyse and optimize routes are: Smartmonkey, Routific, Loginext, Stena Line, Pronto, and DHL’s machine learning project.
Pronto is one of my favorites, an app for standardized data exchange on port calls. The self learning model of the app allows shipping companies, agents and terminals to plan, implement and track their activities during a port call. By analyzing such factors as vessel type, cargo type, location, route, sailing speed and other vessels in the vicinity, Pronto powered by AI predicts vessel arrival times in the port.
Also the machine learning project from DHL has definitely captured my attention. The tool predicts air freight time delays. It analyzes 58 different data points and predicts daily transit delays or speedups up to a week in advance. Furthermore, the machine learning model pinpoints the top factors influencing shipment delays.
Though these organisations have fascinating use cases, they all have one big deficit in common: they all focus on only one transport mode. Smartmonkey, Routific, and Loginext all aim to optimize road transport. Stena Line only considers the sea voyage, and Pronto only takes into account the short distance traveled during a port call. DHL uses its machine learning algorithm to analyse the route traveled by airplane.
Time for a holistic system
These use cases drastically impact efficiency gains, and are a great first step but at the same time only answer part of the puzzle. The physical internet consists of a system which is able to answer “How to get from A to B” when A and B have an ocean and multiple countries in between them. It requires multimodal route optimization.
Imagine a system which has the capacity to map out a route taking into account the different possible combinations of transport modes. The system would only require the input of point A and B to come up with the ideal route. Not only would the system configure the ideal route from a warehouse to a port by truck, it would also consider combining or replacing the first mile delivery with rail,or barge. Moreover, it would take into consideration the different sailing schedules of ships and therefore might advice to ship from another port than the one in the vicinity due to a more fitting sailing schedule, taking into account the inland connection. The calculations would happen again in mirror image at the port of destination. The final ETA could then be compared to the ETA when shipping the same goods by plane, which would make it easier to decide whether it’s worth to spent more. If the system was loaded with economic parameters configured by the user it would even be able to make this decision itself.
Moreover,the ideal route doesn’t only have to revolve around the speed of delivery. The most important parameters can vary per shipment. For some the risk of cargo damage might be a crucial parameter, and the system must advice on the safest route to take. Some companies might be interested in the environmental impact of a transport wherefore the software should take into account the carbon emission of different transport modes and advice eco-friendly transportmodes.
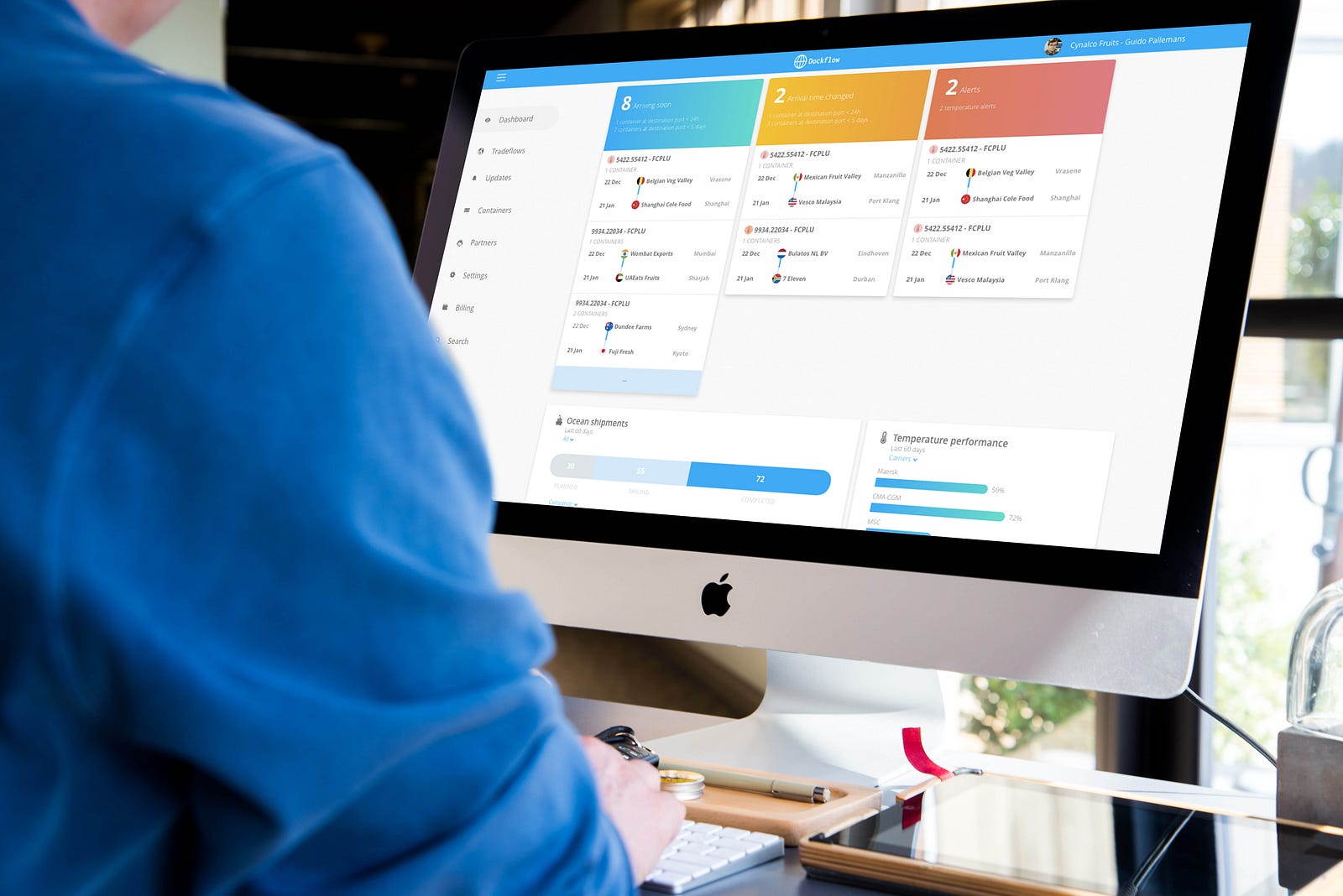
Why?
At Dockflow we all are physical internet enthusiasts. For a matter of fact, this was the reason for the founding of the company: we believe in the creation of a smart system which would allow self-governing supply chains. Our first focus was data governance. To create a smart system data must first be gathered, cleaned and governed, this is how our platform for freight forwarders came into existence.
The next step for us is to start doing smart things with the data we have gathered from tracking & tracing and monitoring temperature on thousands of routes. For that reason we started investigating the possible benefits of artificial intelligence technology. By doing this we discovered a gap in the market: the lack of a multimodal route optimization system, while the question which route to take and which transport modes to consider are fundamental.
As a result, we started working on a new project we named Collie AI which is derived from the smartest dog breed the Border Collie (we are dog lovers). The ultimate goal is to create a system which comes close to the purpose of the physical internet. A system that not only optimizes the route of one transport mode but is able to make intelligent decisions regarding the concatenation of different transport modes. Today these immensely complex decisions are made by humans, which is absurd. Freight forwarders don’t have the time time to analyse each and every route and study the possible transport modes taking into account the time it costs to switch over from one transport mode to the other, the risk of congestion at certain terminals or on the roads, the risk of inadequate water depth for barges etc. Such calculations would require too much time to be efficient. Nevertheless, to date transport depends on freight forwarders and their experience to map out the most efficient way of transport.
We want to change that. Freight forwarders are seen as the architects of transportation and we believe they are missing something in their toolbox. Collie AI will honor the role of a freight forwarder as it will provide them with a decision making framework on which they can base their preference to choose or not choose a certain route for their shipments.
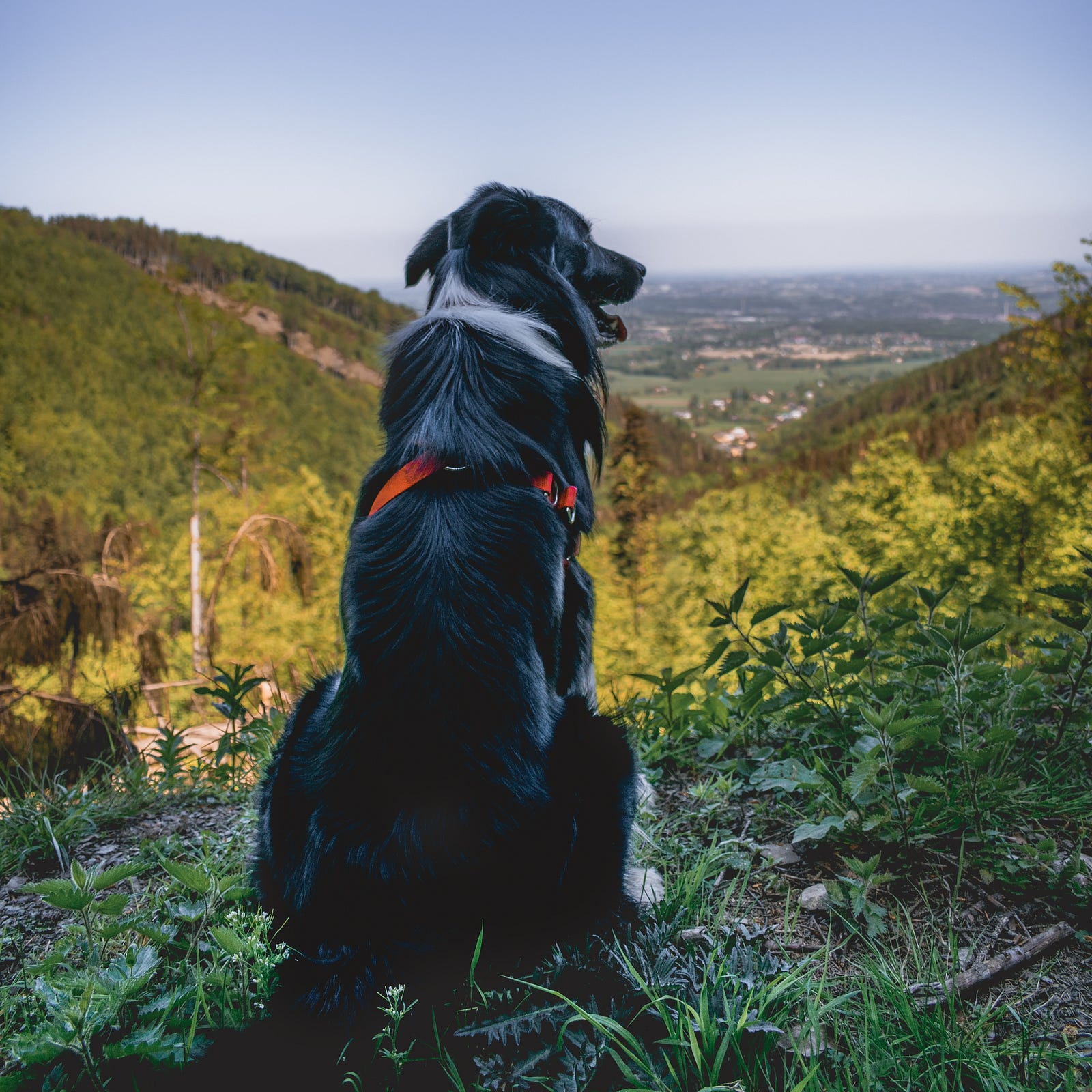
Conclusion
There’s a lot of buzz around the use cases for AI in logistics. Which is understandable as the use cases have already dramatically increased the efficiency of operations. Nevertheless, we are just getting started with this technology and we are nowhere near the true potential of artificial intelligence. On the other hand, the maturity of the technology is growing at a blistering pace. Simultaneously,the process complexity and amounts of data generated by the industry call for an intelligent system to structure, analyse and use this data. In addition, consumer behavior is changing, people want their stuff right now. In today’s world, the speed of delivery matters more than ever.
We are lucky. There’s a lot of potential to make logistics substantially faster/better than it is right now. To date the average dwell time of a container is still between 4 and 7 days.The combination of big-data and artificial intelligence can streamline logistics on a global scale and create the foundation for the Utopian (but achievable) physical internet.
AI has created enormous efficiency gains in single transport mode optimizations, but the technology is ready to take this up a notch. Multimodal route optimization, that’s where we at Dockflow are heading. Nonetheless, realizing this doesn’t mean we are there yet. Future steps will take this even further and allow a system to organize itself on parcel level. Operating on this level means even more possibilities as the systems decides which goods are stuffed in which containers in order to achieve maximum efficiency. How crazy this may seem, I’m sure we will get there faster than we think. As some say the innovation of the shipping container allowed us to (almost) treat the physical atoms of the world like computerized bits. It’s time to create a protocol which makes those bits travel in the most efficient way possible.